Launch and Scale Your AI Organization
You have decided on your first AI initiative, the budget is approved, and it's time to kick off the hiring process. But whom should we hire, and what roles do we need in an AI team? We don’t have enough skilled people available in the market, so as a leader, you should be very clear about hiring the right people for your AI team. This blog covers the various components of an AI system, the skills required for these components, and different strategies for setting up and scaling the team.
Like any product/service, an AI system also has a producer and a consumer. We will cover the producer and the team creating the AI models or systems for others to consume. The blog has been divided into four sections:
- 1. Identify and define the AI components
- 2. Identify roles in a team who can work on these AI components
- 3. Onboarding strategies of the AI team members
- 4. Scaling the AI team
Setting up AI infra, tooling, and deployment is also critical for the AI organization; I will cover it in a separate blog.
As I said at the start of the blog, the first step is defining the business problem, how we are trying to solve it with AI, and what the success criteria are? Don’t start the AI project just because everyone else is doing it. There are different ways of finding out the AI use cases – topic for the future blog.
1. Identify and define the AI components:
An AI system consists of multiple components, and as a leader, you should identify and define these components e.g.
- 1. Data collection and management: Identify, collect, clean, and manage the raw data
- 2. Data preprocessing, cleaning, and transformation: Transform the raw data and make it ready for the AI models
- 3. Model selection and development: choose the AI algorithm and build the model
- 4. Model training and optimization: Train model with data, optimize and improve accuracy
- 5. Model validation and testing: Evaluate the model performance and fine tune it based on the real-world data.
- 6. Model deployment & Integration: Deploy the AI model into production and integrate with other systems, as required.
- 7. Model monitoring and continuous learning: Continuous monitoring, retraining and improving accuracy of the model.
- 8. Data Infrastructure: Ensuring the efficiency and performance of the system at scale
- 9.Governance and Ethics: Fairness, transparency, compliance, and responsible AI.
2. Roles in an AI team:
We need a group of engineers who can form an AI team and work on these components to complete the end-to-end system.
- 1. AI Product Manager: This person defines the business objectives and aligns the AI systems' outcomes with the company vision. You may want to add SMEs, domain experts, BA to support the product manager.
- 2. Data Engineers: They design and maintain the data infrastructure, pipeline, and warehouse. They are responsible for collecting and cleaning the data while maintaining its quality and consistency.
- 3. Data Scientists: Transform the data, design AI models, conduct experiments, and extract insights.
- 4. ML Engineers (including the MLOps): Develop, test, deploy, optimize, and monitor the models.
- 5. AI Engineers: This includes team members responsible for UI design, testing, Cloud infra management, creating interfaces and API for consumers.
- 6. Ethics, Governance and Compliance: Responsible for fairness, bias mitigation, and regulatory compliance.
Like any product development team, the AI team members should also have the domain expertise to identify, clean, and manage the correct data. Relying solely on the AI Product Manager for this expertise may not be enough.
In the next part of the article, I will cover the examples and case studies of organisations following different team structures.
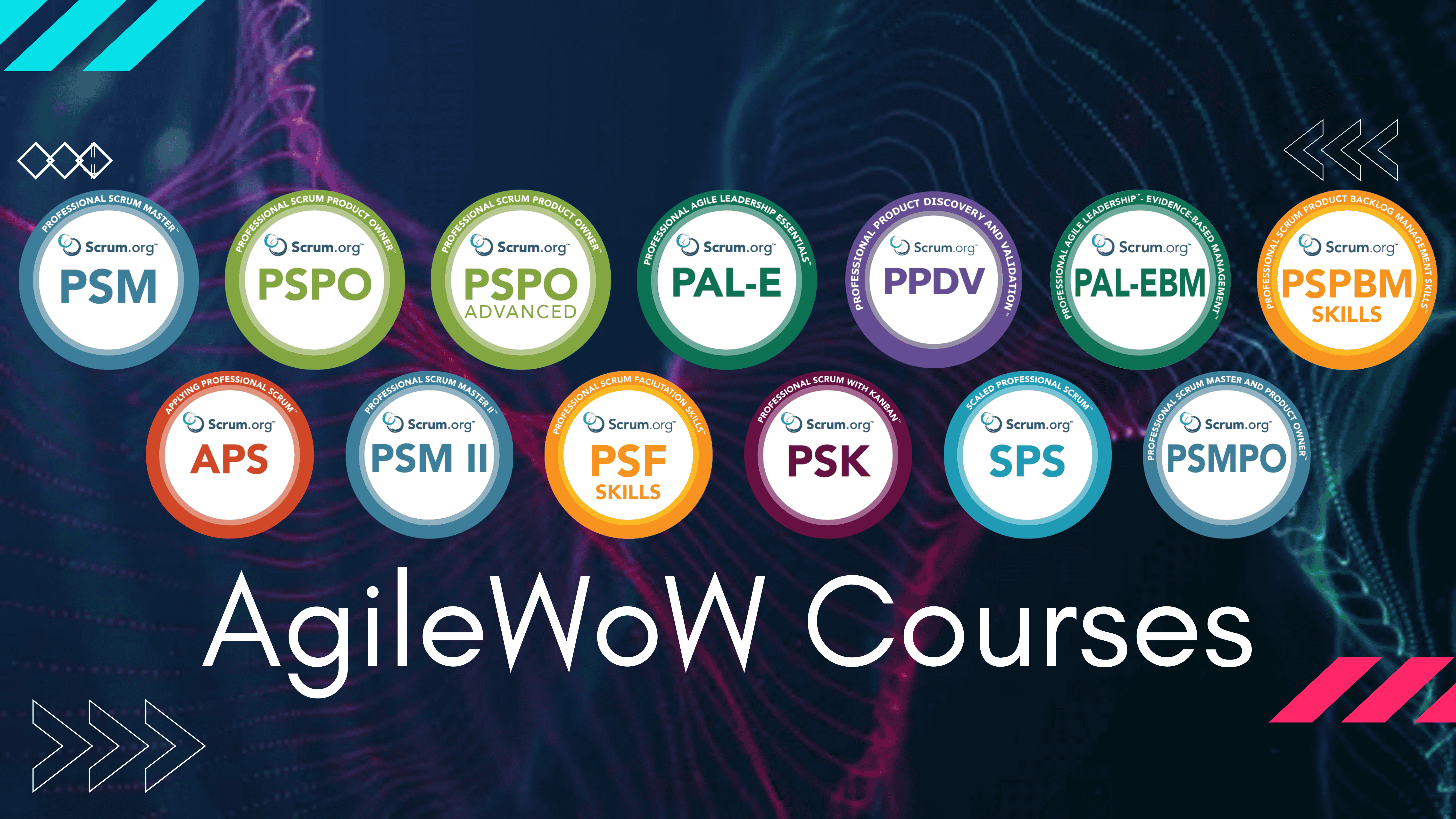
3. Onboarding strategies:
The roles have been identified, so the next step in onboarding these engineers is to build an in-house team, outsource the work, or create a mix of permanent and consultants. Each mode has pros and cons, so the decision is yours depending on your context.
- 1. Outsourcing may be the best option if you lack expertise and need a quick turnaround.
- 2. A hybrid model should work if you want to do POCs or experiments while developing in-house capabilities.
- 3. However, if you have decided on AI as your long-term strategy or are working on a proprietary product or high-security project, setting up an in-house team is the right choice.
In the next part of the article, I will cover examples and case studies of different organisations that follow different onboarding strategies.
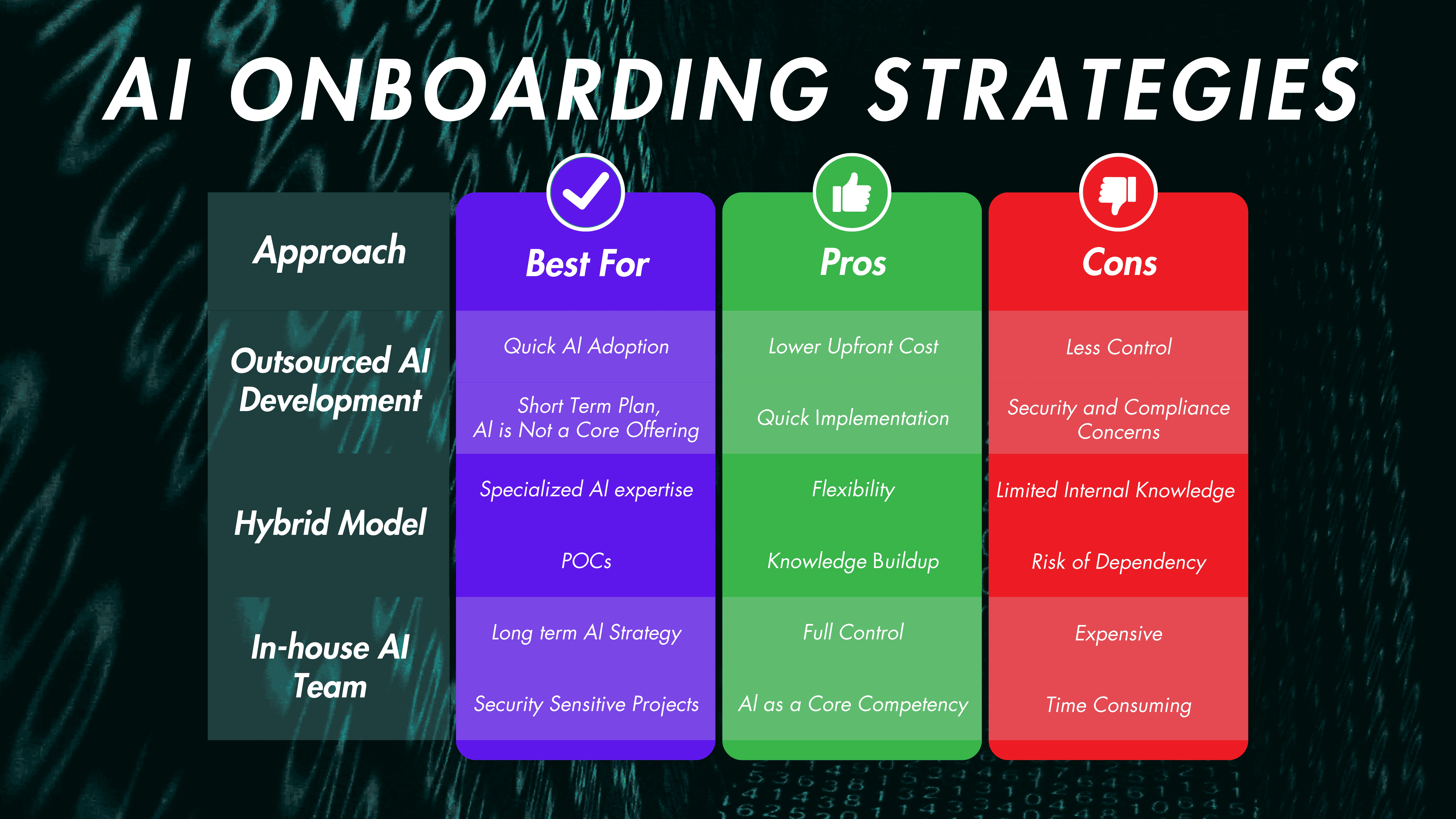
4. Scaling the AI team:
Scaling an AI organization is not just about hiring more people, it is about structuring those people in different teams and business units. The best AI organization don’t just scale AI, they scale it the right way. There are several scaling models, based on your business needs and organization structure you can decide.
- 1. Centralized AI team: This is similar to the traditional CoE, keeping AI under one roof. The CoE manages all AI initiatives and teams across the company request AI solutions from them. This ensures standardization, compliance, and governance across AI projects. As the demand grows the CoE may become a bottleneck.
- 2. Decentralized AI teams: An organization may have multiple business units, and in this approach, each BU has its own AI team working on its specific AI projects. This allows each BU more autonomy and faster innovation per department. But this may create duplication of efforts and inconsistent AI governance.
- 3. Hybrid Model: This is the best of both worlds. A central AI CoE sets standards, infrastructure, and best practices. Each business unit is aligned with the CoE for governance and consistency while focusing on their specific projects and stakeholders.
- 4. AI as a Platform: AI experts build re-usable AI models, APIs, and tools; business teams integrate these capabilities into their workflows without needing much AI expertise. This requires high AI infrastructure investment and strong platform engineering expertise.
Business Need | Recommended Scaling Model |
---|---|
Strong governance, compliance and security | Centralized AI team (CoE) |
Faster execution and innovation | Decentralized AI teams |
Balancing control and speed | Hybrid model |
Services for Business Units | AI as a platform |
Key takeaways for leaders:
- 1. The AI initiative should start with the business objective in focus
- 2. AI teams should align with the business goals.
- 3. Start with small, focused AI team before scaling
- 4. Choose the right scaling model based on your organization needs
- 5. When scaling, do not ignore the ethics, governance, and security concerns.
Are you setting up your first AI team?
Or you are planning to scale your team?